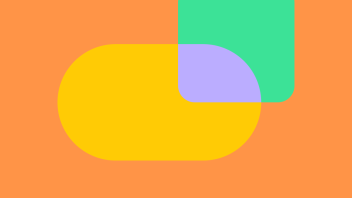
The Mann Whitney U Test (MWU), also known as the Wilcoxon Rank Sum Test and the Mann-Whitney-Wilcoxon Test, continues to be advertised as the go-to test for analyzing non-normally distributed data. In online experimentation it is often touted as the most suitable for analyses of non-binomial metrics with typically non-normal (skewed) distributions such as average […] Read more…