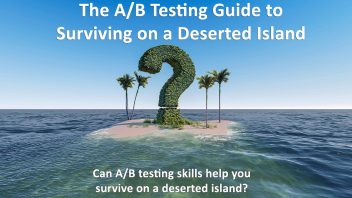
The secluded and isolated deserted island setting has been used as the stage for many hypothetical explanations in economics and philosophy with the scarcity of things that can be developed as resources being a central feature. Scarcity and the need to keep risk low while aiming to improve one’s situation is what make it a […] Read more…