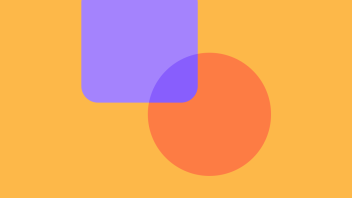
Several charges are commonly thrown at A/B testing while considering it or even after it has become standard practice in a company. They may come from product teams, designers, developers, or management, and can be summed up like this: A good way to address these and to make the business case for experimentation is to […] Read more…