A central feature of sequential testing is the idea of stopping “early”, as in “earlier compared to an equivalent fixed-sample size test”. This allows running A/B tests with fewer users and in a shorter amount of time while adhering to the targeted error guarantees.
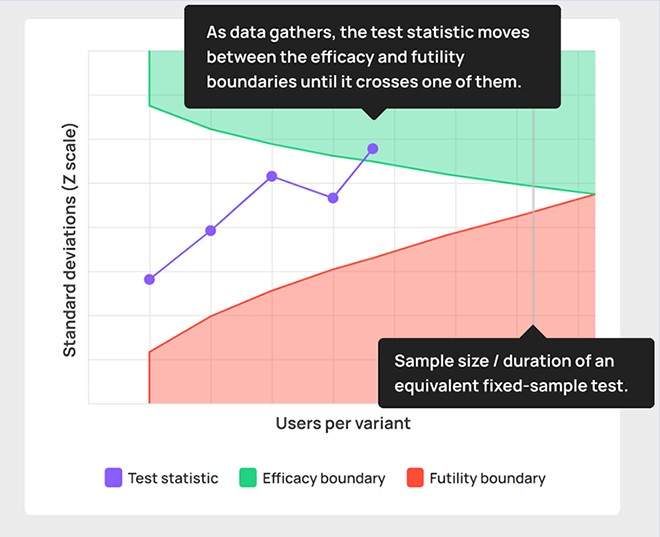
For example, a test may be planned with a maximum duration of eight weeks and a maximum sample size of 100,000 users, but the outcome statistic may indicate for it to be stopped on week four after having observed just 50,000 users.
Sequential tests and testing velocity
Upon seeing the above or upon hearing that the real-world edge of AGILE sequential testing over fixed-sample tests is estimated in a recent meta-analysis to be around 28%, one may focus on the ability to run shorter A/B tests for its own sake.
Through this lens sequential testing is seen as mainly offering improved testing speed (or velocity), meaning the number of tests performed per unit time is, e.g. ten tests per month. A “testing pipeline” with a given maximum throughput capacity is imagined and the benefit of sequential testing is seen as primarily resulting in increased testing capacity, or how soon one can launch the next A/B test.
For experimenters sharing that understanding, sequential testing does not seem to offer that much and proposing its adoption may lead to responses like:
- We are already running the maximum number of tests we are capable of coming up with in any given timeframe, so why would we want the ability to run even more?
- Why would we want to end a test sooner, given the next one may not be ready yet?
- Users are available anyways and including more of them costs next to nothing, so why rush it?
Unfortunately, a focus on testing velocity may result in missing the main value proposition of sequential tests which is about test efficiency in terms of a risk-adjusted Return on Investment (ROI).
Sequential testing improves business results
Similar to how the value of A/B testing is in delivering a positive marginal risk-adjusted return* versus implementing a change without testing, the primary benefit of sequential testing is in improving the risk-adjusted returns* of online experimentation versus fixed-sample tests. This is why the white paper introducing AGILE sequential testing is titled “Efficient A/B Testing in Conversion Rate Optimization: The AGILE Statistical Method” and also why the article which introduced it and explained its virtues is called Improving ROI in A/B Testing: the AGILE AB Testing Approach.
The main utility of sequential tests is a direct effect of their high probability that tests will be stopped sooner on average, no matter what the true effect is. This is especially important when the effect is very good so a variant can be implemented sooner, or when the effect is not good enough to warrant further exposure of users to potentially loss-making experiences.
Faster tests mean smaller loses
Stopping a test early means not exposing 50% (in a simple A/B test) or a larger percentage (in an A/B/N test) of users to a change which is likely resulting in missed revenue for the business. If you stop a test 40% earlier than if it were a fixed-sample test, and the variant had a true negative effect of -10%, then that’s saving the business from a 5% loss of revenue (10% * 50% = 5%) from however many users that 40% is.
If the test was stopped four weeks earlier, then a business making $1mln in revenue per week would be spared a loss of $50,000 due to unrealized sales. If it were making $10mln in revenue, the avoided losses would amount to $500,000. And so on. Even if the percentages are smaller, the absolute numbers can be quite high with sufficiently high stakes.
If your tests are similar to a recent meta analytical estimate in which half of the tests had a negative true effect, then sequential testing would be sparing lost revenue in half of them.
Shorter tests result in larger gains
The ability to stop earlier also results in larger gains if the true effect of the change is positive. Stopping, for example, 40% faster means that the up to 50% users allocated to the control group of a test can start experiencing the beneficial change now instead of in a number of days or weeks. This directly results in a revenue increase for the business.
The math is very similar to that of the avoided losses. For a business with $1mln in weekly revenue, stopping an A/B test in which the true effect is +2% four weeks early means 2% * 50% * 1mln * 4 = $40,000 in additional revenue compared to running a fixed-sample test. If the business in question had $10mln weekly revenue, then the additional revenue gained from stopping early would be $400,000.
Both of the above scenarios show why thinking that including more users in a test costs next to nothing is so dangerous. It can be very far from the truth in case of a non-zero true effect.
See this in action
The all-in-one A/B testing statistics solution
Business results drive the adoption of sequential testing
Given how sequential testing improves business returns, it is no wonder that most advanced experimentation teams employ sequential tests of one kind of another. Not doing so is just too expensive.
Not running sequential tests results in unnecessarily high cost of testing.
Not all sequential tests are made equal, however, and they differ on the efficiency that they deliver. The AGILE sequential method with its futility stopping bounds which limits unnecessary exposure to money-losing user experiences is especially suitable for achieving optimal ROI from testing. AGILE tests almost always result in a greater marginal value of testing when compared to fixed-sample tests. You can start using AGILE immediately by signing up for Analytics Toolkit.
Group-sequential tests have recently seen traction with other testing platforms as well. For example, if you are looking for a fully-fledged in-house A/B testing platform with support of AGILE sequential testing, you should consider A/B Smartly’s excellent product offering. I’m actively involved in advising their team on the implementation details.
Takeaways
The primary utility of sequential methods is in delivering an increased return on investment from testing. It comes through stopping A/B tests earlier than their fixed-sample counterparts, on average, realizing larger gains when the true effect is positive and incurring smaller losses from exposure of users to inferior test variants. The above greatly reduces the business cost of running tests and improves the risk-adjusted returns of testing.
In case there are real or perceived limits on test velocity, sequential testing will also help increase test velocity, but it is a mere side-effect of the lower average sample sizes.
* To learn more about how to quantify the marginal value provided by testing, consider the detailed exploration of the costs and benefits involved in A/B testing and the comprehensive framework for achieving optimal balance of risk and reward in an A/B test that I have proposed. The most complete picture is available in the chapter on optimal significance thresholds and sample sizes in “Statistical Methods in Online A/B Testing” (2019). Notably, all of the above are not merely abstract ideas as they have been implemented since 2017 and are currently embedded in Analytics Toolkit’s A/B testing hub.